RandomOverSampler#
- class imblearn.over_sampling.RandomOverSampler(*, sampling_strategy='auto', random_state=None, shrinkage=None)[source]#
Class to perform random over-sampling.
Object to over-sample the minority class(es) by picking samples at random with replacement. The bootstrap can be generated in a smoothed manner.
Read more in the User Guide.
- Parameters:
- sampling_strategyfloat, str, dict or callable, default=’auto’
Sampling information to resample the data set.
When
float
, it corresponds to the desired ratio of the number of samples in the minority class over the number of samples in the majority class after resampling. Therefore, the ratio is expressed as \(\alpha_{os} = N_{rm} / N_{M}\) where \(N_{rm}\) is the number of samples in the minority class after resampling and \(N_{M}\) is the number of samples in the majority class.Warning
float
is only available for binary classification. An error is raised for multi-class classification.When
str
, specify the class targeted by the resampling. The number of samples in the different classes will be equalized. Possible choices are:'minority'
: resample only the minority class;'not minority'
: resample all classes but the minority class;'not majority'
: resample all classes but the majority class;'all'
: resample all classes;'auto'
: equivalent to'not majority'
.When
dict
, the keys correspond to the targeted classes. The values correspond to the desired number of samples for each targeted class.When callable, function taking
y
and returns adict
. The keys correspond to the targeted classes. The values correspond to the desired number of samples for each class.
- random_stateint, RandomState instance, default=None
Control the randomization of the algorithm.
If int,
random_state
is the seed used by the random number generator;If
RandomState
instance, random_state is the random number generator;If
None
, the random number generator is theRandomState
instance used bynp.random
.
- shrinkagefloat or dict, default=None
Parameter controlling the shrinkage applied to the covariance matrix. when a smoothed bootstrap is generated. The options are:
if
None
, a normal bootstrap will be generated without perturbation. It is equivalent toshrinkage=0
as well;if a
float
is given, the shrinkage factor will be used for all classes to generate the smoothed bootstrap;if a
dict
is given, the shrinkage factor will specific for each class. The key correspond to the targeted class and the value is the shrinkage factor.
The value needs of the shrinkage parameter needs to be higher or equal to 0.
Added in version 0.8.
- Attributes:
- sampling_strategy_dict
Dictionary containing the information to sample the dataset. The keys corresponds to the class labels from which to sample and the values are the number of samples to sample.
- sample_indices_ndarray of shape (n_new_samples,)
Indices of the samples selected.
Added in version 0.4.
- shrinkage_dict or None
The per-class shrinkage factor used to generate the smoothed bootstrap sample. When
shrinkage=None
a normal bootstrap will be generated.Added in version 0.8.
- n_features_in_int
Number of features in the input dataset.
Added in version 0.9.
- feature_names_in_ndarray of shape (
n_features_in_
,) Names of features seen during
fit
. Defined only whenX
has feature names that are all strings.Added in version 0.10.
See also
BorderlineSMOTE
Over-sample using the borderline-SMOTE variant.
SMOTE
Over-sample using SMOTE.
SMOTENC
Over-sample using SMOTE for continuous and categorical features.
SMOTEN
Over-sample using the SMOTE variant specifically for categorical features only.
SVMSMOTE
Over-sample using SVM-SMOTE variant.
ADASYN
Over-sample using ADASYN.
KMeansSMOTE
Over-sample applying a clustering before to oversample using SMOTE.
Notes
Supports multi-class resampling by sampling each class independently. Supports heterogeneous data as object array containing string and numeric data.
When generating a smoothed bootstrap, this method is also known as Random Over-Sampling Examples (ROSE) [1].
Warning
Since smoothed bootstrap are generated by adding a small perturbation to the drawn samples, this method is not adequate when working with sparse matrices.
References
[1]G Menardi, N. Torelli, “Training and assessing classification rules with imbalanced data,” Data Mining and Knowledge Discovery, 28(1), pp.92-122, 2014.
Examples
>>> from collections import Counter >>> from sklearn.datasets import make_classification >>> from imblearn.over_sampling import RandomOverSampler >>> X, y = make_classification(n_classes=2, class_sep=2, ... weights=[0.1, 0.9], n_informative=3, n_redundant=1, flip_y=0, ... n_features=20, n_clusters_per_class=1, n_samples=1000, random_state=10) >>> print('Original dataset shape %s' % Counter(y)) Original dataset shape Counter({1: 900, 0: 100}) >>> ros = RandomOverSampler(random_state=42) >>> X_res, y_res = ros.fit_resample(X, y) >>> print('Resampled dataset shape %s' % Counter(y_res)) Resampled dataset shape Counter({0: 900, 1: 900})
Methods
fit
(X, y, **params)Check inputs and statistics of the sampler.
fit_resample
(X, y, **params)Resample the dataset.
get_feature_names_out
([input_features])Get output feature names for transformation.
Get metadata routing of this object.
get_params
([deep])Get parameters for this estimator.
set_params
(**params)Set the parameters of this estimator.
- fit(X, y, **params)[source]#
Check inputs and statistics of the sampler.
You should use
fit_resample
in all cases.- Parameters:
- X{array-like, dataframe, sparse matrix} of shape (n_samples, n_features)
Data array.
- yarray-like of shape (n_samples,)
Target array.
- Returns:
- selfobject
Return the instance itself.
- fit_resample(X, y, **params)[source]#
Resample the dataset.
- Parameters:
- X{array-like, dataframe, sparse matrix} of shape (n_samples, n_features)
Matrix containing the data which have to be sampled.
- yarray-like of shape (n_samples,)
Corresponding label for each sample in X.
- Returns:
- X_resampled{array-like, dataframe, sparse matrix} of shape (n_samples_new, n_features)
The array containing the resampled data.
- y_resampledarray-like of shape (n_samples_new,)
The corresponding label of
X_resampled
.
- get_feature_names_out(input_features=None)[source]#
Get output feature names for transformation.
- Parameters:
- input_featuresarray-like of str or None, default=None
Input features.
If
input_features
isNone
, thenfeature_names_in_
is used as feature names in. Iffeature_names_in_
is not defined, then the following input feature names are generated:["x0", "x1", ..., "x(n_features_in_ - 1)"]
.If
input_features
is an array-like, theninput_features
must matchfeature_names_in_
iffeature_names_in_
is defined.
- Returns:
- feature_names_outndarray of str objects
Same as input features.
- get_metadata_routing()[source]#
Get metadata routing of this object.
Please check User Guide on how the routing mechanism works.
- Returns:
- routingMetadataRequest
A
MetadataRequest
encapsulating routing information.
- get_params(deep=True)[source]#
Get parameters for this estimator.
- Parameters:
- deepbool, default=True
If True, will return the parameters for this estimator and contained subobjects that are estimators.
- Returns:
- paramsdict
Parameter names mapped to their values.
- set_params(**params)[source]#
Set the parameters of this estimator.
The method works on simple estimators as well as on nested objects (such as
Pipeline
). The latter have parameters of the form<component>__<parameter>
so that it’s possible to update each component of a nested object.- Parameters:
- **paramsdict
Estimator parameters.
- Returns:
- selfestimator instance
Estimator instance.
Examples using imblearn.over_sampling.RandomOverSampler
#
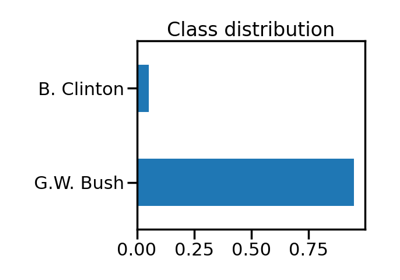
Benchmark over-sampling methods in a face recognition task
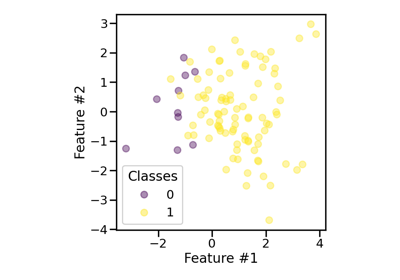
Effect of the shrinkage factor in random over-sampling