BalancedBatchGenerator#
- class imblearn.keras.BalancedBatchGenerator(X, y, *, sample_weight=None, sampler=None, batch_size=32, keep_sparse=False, random_state=None)[source]#
Create balanced batches when training a keras model.
Create a keras
Sequence
which is given tofit
. The sampler defines the sampling strategy used to balance the dataset ahead of creating the batch. The sampler should have an attributesample_indices_
.Added in version 0.4.
- Parameters:
- Xndarray of shape (n_samples, n_features)
Original imbalanced dataset.
- yndarray of shape (n_samples,) or (n_samples, n_classes)
Associated targets.
- sample_weightndarray of shape (n_samples,)
Sample weight.
- samplersampler object, default=None
A sampler instance which has an attribute
sample_indices_
. By default, the sampler used is aRandomUnderSampler
.- batch_sizeint, default=32
Number of samples per gradient update.
- keep_sparsebool, default=False
Either or not to conserve or not the sparsity of the input (i.e.
X
,y
,sample_weight
). By default, the returned batches will be dense.- random_stateint, RandomState instance or None, default=None
Control the randomization of the algorithm:
If int,
random_state
is the seed used by the random number generator;If
RandomState
instance, random_state is the random number generator;If
None
, the random number generator is theRandomState
instance used bynp.random
.
- Attributes:
- sampler_sampler object
The sampler used to balance the dataset.
- indices_ndarray of shape (n_samples, n_features)
The indices of the samples selected during sampling.
Examples
>>> from sklearn.datasets import load_iris >>> iris = load_iris() >>> from imblearn.datasets import make_imbalance >>> class_dict = dict() >>> class_dict[0] = 30; class_dict[1] = 50; class_dict[2] = 40 >>> X, y = make_imbalance(iris.data, iris.target, sampling_strategy=class_dict) >>> import tensorflow >>> y = tensorflow.keras.utils.to_categorical(y, 3) >>> model = tensorflow.keras.models.Sequential() >>> model.add( ... tensorflow.keras.layers.Dense( ... y.shape[1], input_dim=X.shape[1], activation='softmax' ... ) ... ) >>> model.compile(optimizer='sgd', loss='categorical_crossentropy', ... metrics=['accuracy']) >>> from imblearn.keras import BalancedBatchGenerator >>> from imblearn.under_sampling import NearMiss >>> training_generator = BalancedBatchGenerator( ... X, y, sampler=NearMiss(), batch_size=10, random_state=42) >>> callback_history = model.fit(training_generator, epochs=10, verbose=0)
Methods
Method called at the beginning of every epoch.
Method called at the end of every epoch.
- property num_batches#
Number of batches in the PyDataset.
- Returns:
The number of batches in the PyDataset or
None
to indicate that the dataset is infinite.
Examples using imblearn.keras.BalancedBatchGenerator
#
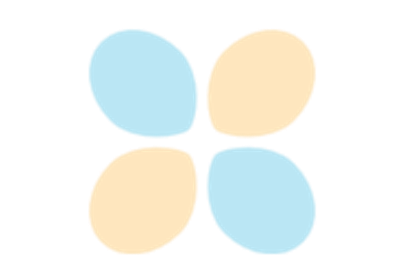
Porto Seguro: balancing samples in mini-batches with Keras