FunctionSampler#
- class imblearn.FunctionSampler(*, func=None, accept_sparse=True, kw_args=None, validate=True)[source]#
Construct a sampler from calling an arbitrary callable.
Read more in the User Guide.
- Parameters:
- funccallable, default=None
The callable to use for the transformation. This will be passed the same arguments as transform, with args and kwargs forwarded. If func is None, then func will be the identity function.
- accept_sparsebool, default=True
Whether sparse input are supported. By default, sparse inputs are supported.
- kw_argsdict, default=None
The keyword argument expected by
func
.- validatebool, default=True
Whether or not to bypass the validation of
X
andy
. Turning-off validation allows to use theFunctionSampler
with any type of data.Added in version 0.6.
- Attributes:
- sampling_strategy_dict
Dictionary containing the information to sample the dataset. The keys corresponds to the class labels from which to sample and the values are the number of samples to sample.
- n_features_in_int
Number of features in the input dataset.
Added in version 0.9.
- feature_names_in_ndarray of shape (
n_features_in_
,) Names of features seen during
fit
. Defined only whenX
has feature names that are all strings.Added in version 0.10.
See also
sklearn.preprocessing.FunctionTransfomer
Stateless transformer.
Notes
See Customized sampler to implement an outlier rejections estimator
Examples
>>> import numpy as np >>> from sklearn.datasets import make_classification >>> from imblearn import FunctionSampler >>> X, y = make_classification(n_classes=2, class_sep=2, ... weights=[0.1, 0.9], n_informative=3, n_redundant=1, flip_y=0, ... n_features=20, n_clusters_per_class=1, n_samples=1000, random_state=10)
We can create to select only the first ten samples for instance.
>>> def func(X, y): ... return X[:10], y[:10] >>> sampler = FunctionSampler(func=func) >>> X_res, y_res = sampler.fit_resample(X, y) >>> np.all(X_res == X[:10]) True >>> np.all(y_res == y[:10]) True
We can also create a specific function which take some arguments.
>>> from collections import Counter >>> from imblearn.under_sampling import RandomUnderSampler >>> def func(X, y, sampling_strategy, random_state): ... return RandomUnderSampler( ... sampling_strategy=sampling_strategy, ... random_state=random_state).fit_resample(X, y) >>> sampler = FunctionSampler(func=func, ... kw_args={'sampling_strategy': 'auto', ... 'random_state': 0}) >>> X_res, y_res = sampler.fit_resample(X, y) >>> print(f'Resampled dataset shape {sorted(Counter(y_res).items())}') Resampled dataset shape [(0, 100), (1, 100)]
Methods
fit
(X, y)Check inputs and statistics of the sampler.
fit_resample
(X, y)Resample the dataset.
get_feature_names_out
([input_features])Get output feature names for transformation.
Get metadata routing of this object.
get_params
([deep])Get parameters for this estimator.
set_params
(**params)Set the parameters of this estimator.
- fit(X, y)[source]#
Check inputs and statistics of the sampler.
You should use
fit_resample
in all cases.- Parameters:
- X{array-like, dataframe, sparse matrix} of shape (n_samples, n_features)
Data array.
- yarray-like of shape (n_samples,)
Target array.
- Returns:
- selfobject
Return the instance itself.
- fit_resample(X, y)[source]#
Resample the dataset.
- Parameters:
- X{array-like, sparse matrix} of shape (n_samples, n_features)
Matrix containing the data which have to be sampled.
- yarray-like of shape (n_samples,)
Corresponding label for each sample in X.
- Returns:
- X_resampled{array-like, sparse matrix} of shape (n_samples_new, n_features)
The array containing the resampled data.
- y_resampledarray-like of shape (n_samples_new,)
The corresponding label of
X_resampled
.
- get_feature_names_out(input_features=None)[source]#
Get output feature names for transformation.
- Parameters:
- input_featuresarray-like of str or None, default=None
Input features.
If
input_features
isNone
, thenfeature_names_in_
is used as feature names in. Iffeature_names_in_
is not defined, then the following input feature names are generated:["x0", "x1", ..., "x(n_features_in_ - 1)"]
.If
input_features
is an array-like, theninput_features
must matchfeature_names_in_
iffeature_names_in_
is defined.
- Returns:
- feature_names_outndarray of str objects
Same as input features.
- get_metadata_routing()[source]#
Get metadata routing of this object.
Please check User Guide on how the routing mechanism works.
- Returns:
- routingMetadataRequest
A
MetadataRequest
encapsulating routing information.
- get_params(deep=True)[source]#
Get parameters for this estimator.
- Parameters:
- deepbool, default=True
If True, will return the parameters for this estimator and contained subobjects that are estimators.
- Returns:
- paramsdict
Parameter names mapped to their values.
- set_params(**params)[source]#
Set the parameters of this estimator.
The method works on simple estimators as well as on nested objects (such as
Pipeline
). The latter have parameters of the form<component>__<parameter>
so that it’s possible to update each component of a nested object.- Parameters:
- **paramsdict
Estimator parameters.
- Returns:
- selfestimator instance
Estimator instance.
Examples using imblearn.FunctionSampler
#
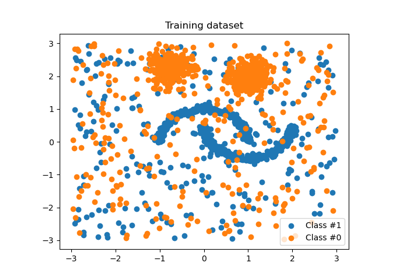
Customized sampler to implement an outlier rejections estimator
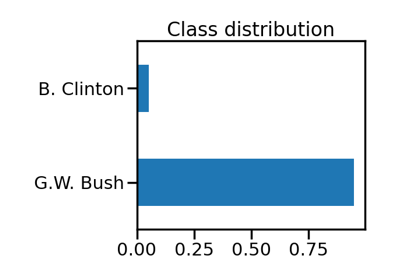
Benchmark over-sampling methods in a face recognition task